Anaconda Accelerates AI Development and Deployment with NVIDIA CUDA Toolkit
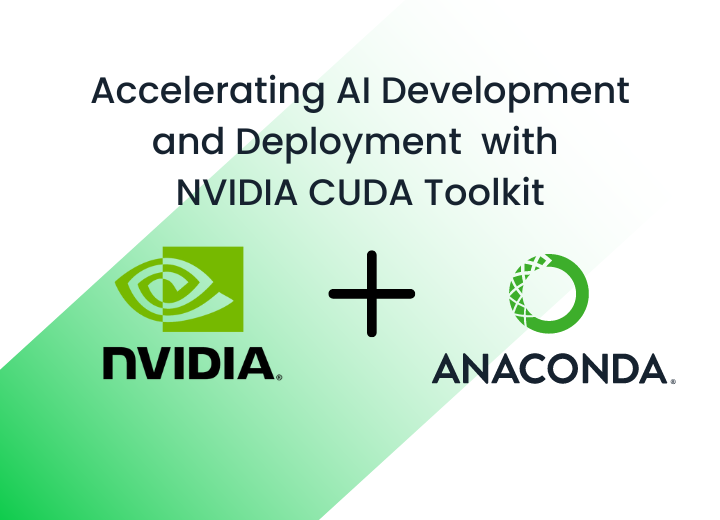
AI adoption has reached critical mass: 99% of organizations are either using AI or actively exploring it, with nearly half in the scaling phase, according to Enterprise Strategy Group research. Yet alongside this growth, risks persist: hallucinations, unscalable deployments, shadow AI, and security vulnerabilities that threaten even promising projects.
“Enterprises need to be really smart about AI adoption because they will sleep-walk themselves into a massive amount of regulatory and implementation hurdles,” said Peter Wang, Anaconda’s co-founder and chief AI officer, during a recent webinar with ESG’s principal analyst, Mark Beccue.
In this blog, I explore key takeaways from their conversation and share insights from our recent “2025 State of Enterprise Open Source AI” report.
“Enterprises are adopting open-weight models for good reason,” Wang said. “They want to control their own destiny.”
What did Wang mean by open-weight models? Well, when most companies talk about open source AI, what they actually mean is open-weight models – pre-trained, publicly available AI models that anyone can download and use.
“Open source models should include the training data that was used to train the model, and nobody wants to talk about their training data. Nobody,” Wang emphasized.
While open-weight models conveniently allow organizations to start harnessing the power of AI, they still represent a “black box” system because the training data isn’t available, preventing organizations from fully interpreting how it processes information or produces outputs. Without proper model interpretability, you’re essentially flying blind, hoping the AI won’t suddenly change its behavior or produce unpredictable results that could damage your brand’s credibility.
“A lot of AI vendors are motivated by an arms race right now,” Wang said. “Who has the safest and most performant model? They change and tweak that stuff all the time. It feels like you don’t own your own destiny. That’s why enterprises love to bring all the stuff in-house. They don’t want to upload data out into the cloud. They have no idea if some hot-shot Silicon Valley startup is still going to be around next week. It makes all the sense in the world.”
This, Wang said, is the real value of open source for enterprises, the ability to control your AI destiny. With open source, organizations gain unprecedented transparency, flexibility, and customization options that proprietary solutions can’t match. They can tailor models to their specific needs while maintaining full visibility into how they work. To better understand the state of enterprise open-source AI adoption, we recently surveyed 100 IT decision-makers. Here are some of our key findings.
In our survey, we found a clear throughline of open source AI’s benefits:
Open source solutions offer increased transparency, speed, and customization, but our survey also surfaced some important considerations to keep in mind when implementing open-source solutions. Like any powerful technology, open-source AI works best with thoughtful implementation and proper governance.
For instance, 32% of respondents reported accidental exposure to security vulnerabilities stemming from their use of open source solutions. Likewise, 38% faced challenges in managing model drift, and 36% cited computational resource limitations for training their models.
These issues around security and scalability are tough to navigate. Thankfully, Wang and Beccue discussed how to approach them and build a lasting AI foundation.
Imagine building an ancient city without walls. Invaders would strike with ease and take or destroy pretty much everything. Building and implementing an open source AI infrastructure is similar. Without the right precautions, every AI project risks becoming a liability.
“It’s easy to see all these great open source capabilities as a sort of free puppy,” Wang said. “It seems like a great deal, but once you bring the puppy inside, you realize there are a lot of things you need to actually enjoy it.”
As a practitioner and innovator, Wang learned the value — and vulnerabilities — of open source technology first-hand. “For enterprises to turn [open source software] into a piece of infrastructural technology they can rely on for years, there are a lot of things they need that the open-source ecosystem will not deliver to them.”
These kinds of security concerns are exactly why it can help to have a trusted platform and partner that curates the right models and makes sure everything is safe and compliant in your open source ecosystem. Anaconda, for example, has spent years helping enterprises manage and secure their open source supply chains.
“We created the data science revolution around Python,” Wang said, “it’s a very natural extension to go from that to helping enterprises in their adoption and maturity process as they lean into open source AI.”
10% of organizations accidentally installed malicious code during their use of open source components. 60% of affected respondents described the impact as very or extremely significant.
After securing your environment, you need to implement the right infrastructure, which requires two key elements: hardware and culture.
“AI workloads are very similar to ML and data science in that they’re extremely bursty and require exotic hardware. They can be extremely memory-intensive and expensive. So, small and mid-size enterprises adopting this technology need to understand that their compute footprint is going to change quite a bit.”
The cultural shift is equally challenging. “The biggest gap is that perspective gap of who’s actually building new kinds of software systems and new information processing systems,” Wang said. “It’s going to come from all the lines of business. They’re going to be building applications and have new kinds of things they want to do with the business data. And they’re going to have executive support. This is the world that’s coming, and I think that’s going to be really a hard change for a lot of businesses to make.”
Why is this so difficult? Consider how organizations in the early 2000s would hire a data scientist only to make them wait months for data access. Now imagine this conservatism multiplied across departments suddenly needing unprecedented data access. Overcoming this cultural resistance is imperative for any successful AI initiative
With security and infrastructure in place, the final challenge is building momentum inside your organization. Innovation starts with people who understand AI’s potential and limitations.
Fostering AI literacy across your organization is critical for adoption. As Jess Haberman explains in Unite.AI, organizations need “upskilling pathways that empower employees to use AI judiciously and responsibly,” creating understanding that supports innovation and addresses resistance.
“Look for areas where you will get the least pushback for moving fast and doing some innovation work,” Wang said. “The biggest impact areas are the ones that are most protected. So, you might have to find new areas where you can be free to innovate, but look for that intentionally and get peer and executive buy-in.”
Wang explained that success here first requires tapping the right talent. “Find the technical people in different business units who want to lean into innovation. Those people need to not only be the innovators who know the best models and best practices, but they also need to be key governance stakeholders.”
80% of data science teams use open source AI and ML tools.
Wang recommends involving multiple perspectives, including legal, compliance, and regulatory. “You’re going to run into these stakeholders anyway, so you might as well find the people who are open as opposed to being a tower of ‘no.’” Early involvement will help you tell a compelling story after your first project, where you can show how you adopted cutting-edge technology safely and responsibly. This will give your movement legs — and pave a “golden path” forward.
“You can’t wait for people to do it for you,” Wang said. “You can’t get innovation paths like this laid out fast enough if you wait for the big central IT folks to roll them out.”
“We are in the beginning stages of this truly cybernetic revolution,” Wang said. The next phase of AI is “about taking those predictions and turning them into actions, then learning from those actions and refining the predictions – AI in the loop at every step. That is the way of the future.”
This transformation will be easier for tech companies already using open source. The challenge is for traditional businesses that must become AI-powered regardless of preference.
“There is not a business in the world that doesn’t have aspects of logistics, production, quality control, or other parts of their processes that couldn’t be improved or made safer with AI,” Wang said. “Over the next 10-15 years, every business in the world is going to transform into a cybernetic business, and every business has to decide how it will build these smarts, what its perspective on safety is, and where it’ll make efficiency gains. All of that starts today with their posture on how much they want to control their own destiny.”
The AI revolution won’t wait. Every business will become a cybernetic business, learning, adapting, and optimizing in real time. The question is: Will you control your AI destiny, or let someone else define it for you?
Want to learn more? Watch the full webinar, “Understanding the State of Enterprise Open Source AI & Adoption Challenges,” and read our full report: “The 2025 State of Enterprise Open Source AI.”