Enterprise Data Science, Machine Learning, and AI
Top 10 AI Platform Use Cases For the Enterprise
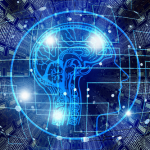
Talk to one of our financial services and banking industry experts to find solutions for your AI journey.