New Release: Anaconda Distribution Now Supporting M1
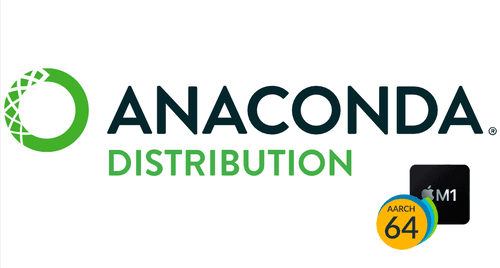
As data science matures, it’s shedding some of its mystique, which is a good thing in many ways. With more organizations seeing returns on their investments in data science capabilities, business leaders are gaining a more realistic view of the field. But while some may have developed a more nuanced understanding of what data science can do, few comprehend how data science actually gets done. So even as its practitioners become essential employees, the field remains a “black box” for many.
This was one of the findings from our 2021 State of Data Science report, in which we asked over 4,000 data practitioners from over 140 countries to name what they see as the biggest myths in data science. The results suggest that the practice and profession sometimes remain opaque for the C-suite and other stakeholders. For data science to continue delivering on its great potential, we must correct faulty assumptions held by organizational leaders.
In this post, we’ll walk through the top myths cited in this year’s State of Data Science report, along with explanations to help dispel them.
Many companies think that quantity should be at the forefront when collecting data. This isn’t surprising, given research and survey processes often teach that large sample sizes will allow for better conclusions. But as more businesses apply analytics to their decision-making, demand is rising for ever-greater volumes of data. Does hoarding mountains of data really improve performance?
Practitioners say no. When asked what the biggest myth in data science is, 31% of respondents said it’s the idea that having access to more data translates to greater accuracy. Another 15% of respondents selected “you need a lot of data” as the top misconception about data science. There are benefits to deep and broad pools of training data, such as solving variance issues. But more data doesn’t necessarily address other issues, like bias, nor can it replace more conventional analysis. Companies with the most advanced data science capabilities already know this.
Where should businesses focus their data efforts, then? As the saying goes, quality over quantity. Instead of asking if it’s enough, organizations should ask if they provide teams with clean, relevant, and useful data for what they seek to model. In fact, enormous amounts of low-quality data may lead to noisy results and poor insights, as shown by disappointing early efforts to deploy AI against COVID-19. Companies would do better and make their data scientists happier if they prioritized stronger data management practices and better communication instead.
After nearly two years of pandemic-disrupted factory floors and snarled supply chains, now coupled with a tight labor market, executives across industries are turning to the promise of automation. Some might consider data science, the foundation for much of today’s automation, as a natural candidate for the next wave of AI-enabled disruption. But that scenario seems unlikely: 33% of respondents in our survey consider the most significant myth to be that data scientists will be replaced by AI soon.
Few data scientists appear worried that machines are coming to replace them. On the contrary, they see an opportunity for AI and automation to help with easily repeatable tasks to free up more resources for work that requires human intervention, interpretation, and problem-solving. Simply put, automation will allow people to develop more complex models or algorithms and spend less time on routine work. It’s no surprise, then, that only 4% of respondents to our survey hold a negative view of AutoML, while 55% have a positive sentiment toward it.
Data science is still an emerging field, and many organizations are only now hiring dedicated data science talent. Data scientists are often grouped in with other “technical” employees within an organization. Compared to a software engineer, it might be tempting to believe that data scientists don’t know how to work with code. But make no mistake: the vast majority of data scientists are programmers, too, just of a slightly different type. Of those surveyed, 19% listed “data scientists don’t know how to code” as the biggest myth about data science.
The difference between a data scientist and a software engineer is how, when, and why they code. For data scientists, Python is typically a fundamental skill in their toolbox for extracting insights from data sets. They’re working with the code of their data pipelines and machine learning models to query data, engineer features, and build and deploy models. By contrast, software engineers use code primarily for product development, often focusing on infrastructure, automation, testing, and maintenance. Even so, because of the wide variety of skills required to become a software engineer, some skills will eventually overlap with those of data scientists—these groups have more in common than many people realize.
As data scientists continually seek to integrate more effectively with other business units in their organizations, it’s essential to take the time to dispel common myths like these, where feasible. Raising awareness for how data scientists work can help improve everything from the accuracy of model predictions to the quality of candidates recruited to fill open positions.
Are there other data science myths you think need to be dispelled? Tell us by letting us know on Twitter.
Talk to one of our experts to find solutions for your AI journey.