An Introduction to the Seaborn Objects System
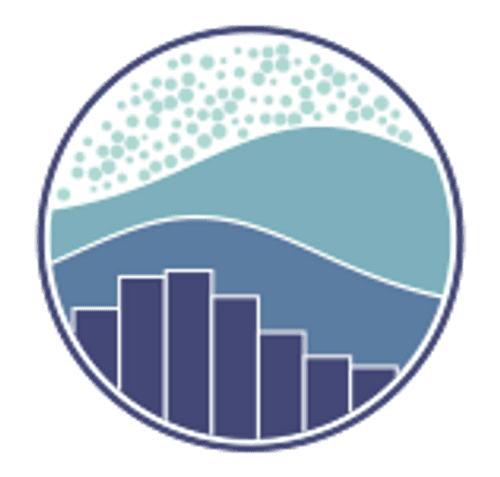
After soliciting information for our 2021 State of Data Science report, the ubiquitous nature of the title “Data Scientist” was immediately apparent. We had over 4,000 respondents, and only 11% of them actually identified themselves as Data Scientists. Another 11% identified as Business Analysts, and the rest of the respondents fell into a multitude of other categories including, Developers, DevOps, MLOps, and more. There’s a lot of crossover amongst these titles, which means they all encompass aspects of what it means to be a Data Scientist.
At a high level, Data Scientists are responsible for cleaning, organizing, and generally making sense of large amounts of data; our above-referenced State of Data Science report indicates that data preparation, data cleansing, and reporting are some of the tasks Data Scientists spend the most time on. Of course, day-to-day schedules differ from industry to industry and department to department. And when it comes to industries, the reach of Data Science is vast; Data Scientists work in technology, health care, finance, manufacturing, government, and many other fields.
The actual work of Data Scientists also varies by the departments they touch, as Data Scientists become less and less siloed within their organizations. In Anaconda’s soon-to-be-released 2022 Predictions webinar, Christine Doig, Director of Innovation for Personalized Experiences at Netflix, talks about the integration of Data Scientists into various departments of a company.
“When we started, there was one single kind of Data Scientist,” she says, “and now the role has been integrated into the organization. Now, there’s a lot more specialization, even within a Data Science team. There has also been an expansion beyond what was traditionally purely a Data Science team; for example, at Netflix, we have the role of Algorithms Product Manager. There’s more integration with the design team, with creative teams. I think that has been a shift that we’ve seen in Data Science over the last few years.” No doubt this trend will continue.
One department in which Data Scientists are becoming increasingly present, regardless of the company, is product management. Why? Because they can help position the product team ahead of the market by facilitating evidence-based decision making, experimentation, and innovation. Involvement with the team ensures that Data Scientists are aligned with product and business objectives.
There are several titles that seem to pop up in conjunction with Data Science regularly. Beyond Data Scientists, there are Business Analysts, Data Analysts, and more. So what is the difference between all of these very similar-sounding roles?
We turned to Sheetal Kalburgi, Associate Product Manager at Anaconda, for help answering this question. According to Sheetal, Data Scientists are more technical and statistical. Data Scientists are responsible for tasks like developing complex statistical algorithms that communicate product performance, predict outcomes, design experiments such as A/B testing, and optimize computational operations to name a few. Business Analysts fall on the other side of the technical spectrum. They are more involved in decision-making pertaining to the business, such as growth analysis, target growth, and if and how to get there, and Data Analysts fall somewhere in between. Data Analysts extract meaning from data and communicate it to decision-makers, almost functioning as liaisons between the Data Scientist and Business Analyst. Business Analysts tend to focus on anomalies, trends, and so on to solve business problems keeping the business model in mind, while a Data Scientist employs statistics, machine learning algorithms to be able to communicate a solution to a problem backed with evidence and data.
A fourth role to consider is that of the Data Engineer. Albert DeFusco, Anaconda’s Principal Product Manager, thinks that as more organizations turn to data insights to help make business decisions, the need for Data Engineering will grow rapidly. While data science and data engineering fields are related, often these two fields work in silos. Albert thinks that will change in the coming year as tools and platforms provide more opportunities for merging Data Science and Data Engineering use cases.
Finally, keep in mind that a large majority of Data Scientists are Programmers, too. While some people are under the impression that Data Scientists don’t code, the opposite is true. Anaconda Data Scientist Sophia Yang elaborates on this point in a recent blog post. “Compared to a software engineer,” she says, “it might be tempting to believe that data scientists don’t know how to work with code. But make no mistake: the vast majority of data scientists are programmers, too, just of a slightly different type.” Sophia goes on to say that Data Scientists often use Python to extract insights from data sets. They work with the code of their data pipelines and Machine Learning models to query data, engineer features, and more.
While there’s no one path to becoming a Data Scientist, you may wish to pursue a Bachelor’s degree in math, computer science, or a similar subject. After that, a graduate degree, perhaps specifically in data and/or analytics, is in order. It’s also a good idea to learn more about the industry you are most interested in joining, whether that’s eCommerce, transportation, healthcare, or something else. And of course, beyond industry, you’ll want to consider whether or not there is a particular company you want to work for, like Netflix, Meta AI Research, Wikimedia Foundation, or even Anaconda.
If you don’t end up completing a university degree, there are bootcamps that can put you on track toward becoming a Data Scientist. Codecademy and Kaggle, for example, offer Data Science bootcamps. These types of programs can help you build projects you can share as you build industry connections and hunt for your first professional opportunity.
After establishing a solid foundation, there is plenty of on-the-job learning to be done. This is the point where you can see Machine Learning theories, for example, being implemented. As you advance, consider becoming more specialized while continuing to grow your general knowledge. This will make you more valuable and set you up for long-term success.
Talk to one of our experts to find solutions for your AI journey.