State of Data Science 2022: Paving the Way for Innovation
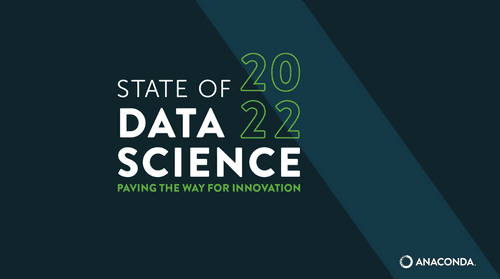
Here’s a truth that I think is critically important, but vastly under-recognized in today’s data-driven world: there is no such thing as “data.”
That might seem like an odd claim for the leader of a data science company to make, so let me explain. What we consider to be “raw” data is in fact information that has already been impacted by models—models that dictate how the data is collected, which data is collected, and the ways in which the collection process itself affects the data. The idea of pure or raw data is a fallacy, as is the practice of treating data as some sort of sacrosanct pure resource like gold or oil; instead, we need to recognize data for the fluid and constraint-dependent representation that it actually is.
For example, think of a photograph. It’s tempting to imagine the raw, unedited image as being a pure data point, or an exact representation of an absolute truth. But consider all the elements of the process that results in that photo: there’s a Bayer matrix, gamma correction, sharpening, stuck pixel, and sensor dust filters, etc. Not to mention, there’s the impact of the exposure time, which is a temporal sampling act in and of itself. Even a raw photo is the product of constraints that inevitably shape the information it contains, which means we can’t take it as a pure form of truth without examining how those constraints have impacted it.
Every single piece of data and every row in a database is the result of a collection system. Filtering, gathering, transforming—so much happens to “raw” input before it becomes usable data. When we look at datasets, we need to understand how much of a model is frozen into them from the outset, in order to get the most value from them.
Of course, this line of thinking can become disorienting or dispiriting quickly; if there is no such thing as pure data, how do we operationalize and generate useful insights from the data we do have? How do we avoid just treading water on this vast ocean, and instead make actual progress toward our goals? And how, if at all, should this understanding about the true nature of data impact the way we approach it?
With the right attitude, this knowledge of the reality of data can actually help you become a more powerful and insightful data user. By understanding the possible limitations or biases of your data, derived from the way it’s been collected or its provenance, you can more intentionally act on insights it delivers. For example, imagine you work for a cell phone service provider and want to survey cell phone users to learn how to improve your product offering. An easy way of selecting survey recipients could be to collect names from billing statements, determine the number of participants you want, and then randomly send surveys to that many names from the list. But what about the fact that for people on family plans, only one name is likely listed as the responsible party for billing, and that person is most likely an adult? You could be missing out on insight from teenage or young adult users, which might make up a sizable portion of your user base because the methodology for data collection was constrained. By thinking through the “frozen models” that have impacted your supposedly “raw” data, you can make more measured and informed decisions from it.
Considering the limitations of your data and the assumptions baked into it isn’t only important for making more informed choices; it’s also a key element of being an ethically responsible data practitioner. The way data is collected can introduce harmful biases, which means AI models or business decisions driven by that data can be biased, too. It’s important to ask questions like “How did we choose which data to collect?” and “Which data points may have been excluded as a result?” when gathering datasets in order to account for—and mitigate—any possible harmful biases. Many of these biases are unintentionally frozen into data, so every dataset should be examined through this lens, even if you think it is unbiased and fair.
There are many different parameters you could tweak in your data collection process to try to eliminate biases or counteract limitations, such as collecting more granular datasets, or taking the opposite approach, and gathering wider swaths of information. Knowing the myriad factors that affect “raw” data, how do you settle on a productive path forward? If you want to get the maximum possible value out of your data, I recommend viewing it through the OODA (observe, orient, decide, act) loop of your business, and using that to set the appropriate parameters for data collection.
For instance, take the task of mapping a neighborhood: the location of streets, sidewalks, vacant lots, houses, etc. If my goal is to set up a sprinkler system for large grassy areas, the level of detail I need is different from what I need to deliver a package to a specific house, which is still different from the level I need to be able to fly a drone through an open window of that house. As a business, you could pour endless resources into collecting data with different textures and at different levels of granularity. It’s important to determine at the outset what type of data you need, and then make sure that your collection process is yielding that information—all the while, being aware of potential biases or blind spots that could be baked into the data as a result of the collection process.
In the data science and machine learning world, we view data and models as being much more fluid and complex, rather than as some sort of static artifact. The more businesses and other organizations can embrace this mindset, too, the more benefit they’ll get out of being data-driven.
For a deeper look at this topic, check out this episode from The a16z Podcast, featuring a conversation between Peter and Martin Casado.
Talk to one of our experts to find solutions for your AI journey.