The Abilities and Limitations of ChatGPT
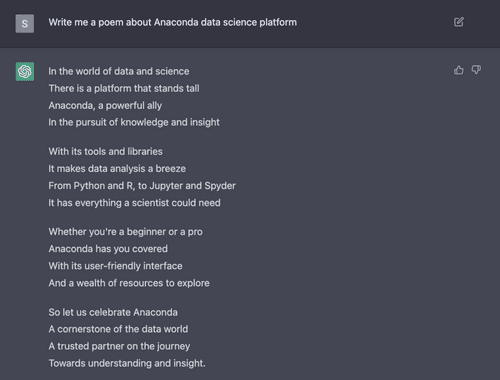
This article was first featured in TechCrunch’s Extra Crunch. You can read the original piece on their website here.
By 2025, 463 exabytes of data will be created each day, according to some estimates. (For perspective, one exabyte of storage could hold 50,000 years of DVD-quality video.) It’s now easier than ever to translate physical and digital actions into data, and businesses of all types have raced to amass as much data as possible in order to gain a competitive edge. However, in our collective infatuation with data (and obtaining more of it), what’s often overlooked is the role that storytelling plays in extracting real value from data.
The reality is that data by itself is insufficient to really influence human behavior. Whether the goal is to improve a business’s bottom line or convince people to stay home amid a pandemic, it’s the narrative that compels action, rather than the numbers alone. As more data is collected and analyzed, communication and storytelling will become even more integral in the data science discipline because of their role in separating the signal from the noise.
Yet this can be an area where data scientists struggle. In Anaconda’s 2020 State of Data Science survey of more than 2,300 data scientists, nearly a quarter of respondents said that their data science or machine learning (ML) teams lacked communication skills. This may be one reason why roughly 40% of respondents said they were able to effectively demonstrate business impact “only sometimes” or “almost never.”
The best data practitioners must be as skilled in storytelling as they are in coding and deploying models—and yes, this extends beyond creating visualizations to accompany reports. Here are some recommendations for how data scientists can situate their results within larger contextual narratives.
While ever-growing datasets help machine learning models better understand the scope of a problem space, more data does not necessarily help with human comprehension. Even for the most left-brain of thinkers, it’s not in our nature to understand large abstract numbers or things like marginal improvements in accuracy. This is why it’s important to include points of reference in your storytelling that make data tangible.
For example, throughout the pandemic, we’ve been bombarded with countless statistics around case counts, death rates, positivity rates, and more. While all of this data is important, tools like interactive maps and conversations around reproduction numbers are more effective than massive data dumps in terms of providing context, conveying risk, and, consequently, helping change behaviors as needed. In working with numbers, data practitioners have a responsibility to provide the necessary structure so that the data can be understood by the intended audience.
Concerningly, only 49% of student respondents in the 2020 State of Data Science survey said that they were learning about data visualization in school. Whether through independent study or as part of a formal curriculum, this is a skillset that data scientists should make time to invest in, as it can improve the reach and efficacy of their work.
Data science doesn’t happen in a vacuum—to effectively use data for problem-solving, data scientists must work in tandem with subject matter experts to understand the problem space and align their hypotheses. While data scientists are responsible for much of the underlying model work and coding, the interpretation of results will be most effective with involvement from subject matter experts to share business or industry-specific context.
Not only can subject matter experts help illuminate hypotheses that data scientists may have overlooked, but they can also ensure that the interpretation of data doesn’t rely on fallacies like “correlation equals causation.” As author Tyler Vigen has humorously pointed out on his website about “Spurious Correlations,” just because two data sets seem correlated doesn’t necessarily mean that we can draw a viable conclusion from them. While the examples he uses—such as the 99% correlation of per capita consumption of margarine and the divorce rate in Maine—may seem obviously fallacious, this can be an easy trap to fall into in business settings in the absence of industry-domain expertise.
For example, a data scientist for an e-commerce service may identify an increase in website traffic on Mondays as an indicator that consumers prefer to shop after weekends, whereas a marketing analyst may point out that the spikes can be explained by recent marketing campaigns falling on the weekends. The analytical chops that data scientists bring will be most effective when presented with the context of business-specific domain knowledge.
Charts and graphs can be a valuable tool, as mentioned earlier, but they can also carry a sense of authority that overshadows the reality that data visualizations or non-visual presentations can be manipulative (see the margarine-and-divorce-rates example above). This is because data is often seen as a fixed source of truth, yet the presentation of data is in fact subjective, largely dependent on who’s interpreting it and how. While data visualization can help identify trends, patterns, or outliers in datasets, it can rarely be used for full analysis and interpretation by itself.
Instead, data analysis needs to be presented with the full context of how the dataset was gathered, what types of analysis were run on it, and where the potential shortfalls may lie. This means asking questions like “Why is this data presented in this way?” and “What kind of trade-offs were made in tuning the parameters for this model?” When presenting their findings, data scientists need to tell this full story of how they arrived at a particular result or insight—doing so not only helps illuminate any potential biases or blind spots, but will also increase confidence over time in data-based decisions. Using data science in a business setting is kind of like eating raw sushi: you feel a lot better about it if you know where the ingredients came from and how they’ve been handled.
Data alone doesn’t spur innovation—rather, it’s data-driven storytelling that helps uncover hidden trends, powers personalization, and streamlines processes. Just as data has the potential to improve decision-making and fuel innovation, data can also create confusion and obscurity. Data practitioners have a growing responsibility to not only analyze data, but also guide their organizations towards harnessing data for effective storytelling.
Talk to one of our experts to find solutions for your AI journey.