Introducing Anaconda Team Edition: Secure Open-Source Data Science for the Enterprise
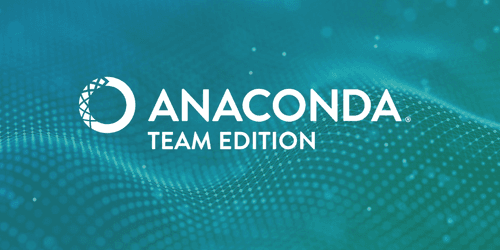
Hassam Mian
4min
From parts suppliers to vehicle manufacturers, service providers to rental car companies, the automotive and related mobility industries stand to gain significantly from implementing machine learning at scale. We see the big automakers investing in proof-of-concept projects at various stages, while disruptors in the field of autonomous driving are trying to build entirely new businesses on a foundation of artificial intelligence and machine learning.
There are huge opportunities for machine learning to improve both processes and products all along the automotive value chain. But where do you focus? And how can you make sure your investments in machine learning aren’t just expensive, “one-and-done” applications? We’ve rounded up four machine learning use cases that can be implemented using open-source technologies and offer long-term value beyond the initial application.
Image recognition and anomaly detection are types of machine learning algorithms that can quickly detect and eliminate faulty parts before they get into the vehicle manufacturing workflow. Parts manufacturers can capture images of each component as it comes off the assembly line, and automatically run those images through a machine learning model to identify any flaws. Highly-accurate anomaly detection algorithms can detect issues down to a fraction of a millimeter. Predictive analytics can be used to evaluate whether a flawed part can be reworked or needs to be scrapped. Eliminating or re-working faulty parts at this point is far less costly than discovering and having to fix them later. It saves on more expensive issues down the line in manufacturing and reduces the risk of costly recalls. It also helps ensure customer safety, satisfaction and retention.
To implement an image recognition and analytics model, the manufacturer needs an accurate dataset containing hundreds or even thousands of parts images, each one tagged with information such as pass, fail, issue A/B/C, etc. The data scientist constructing the model must also have domain expertise regarding allowable tolerances and the potential performance and safety impact of various flaws.
The same approach can be used for all component manufacturing as well as throughout the vehicle assembly line. Image recognition and analytics models can play multiple roles across the automotive value chain — such as recognizing and evaluating tiny variations in tread wear patterns to help develop new and better-performing tires, providing quality control for paint and other finishes, and enabling hazard avoidance for Advanced Driver-Assistance Systems (ADAS) and autonomous driving systems. For this reason, many organizations would realize greater value from an enterprise data science platform, rather than a point solution designed for a single use case.
When an issue arises at any point in the product lifecycle — whether it’s something found early in the manufacturing process or an issue affecting multiple vehicles in the field — organizations scramble to determine the exact cause and how to resolve it. The brand’s reputation (and possibly consumer safety) are at stake.
During the manufacturing phase, identifying the root cause(s) of an issue is a lengthy and painstaking process. Root cause analysis uses massive amounts of testing data, sensor measurements, manufacturer parameters and more. Performed with traditional methods, it’s also incredibly hard.
Root cause analysis for issues in the field isn’t any easier. Today’s vehicles are highly complex, and each driver has unique behavior, maintenance actions and driving conditions. Some issues arise only under very unique circumstances that were unseen in the manufacturing process.
Machine learning techniques can vastly accelerate root cause analysis and speed resolution. Anomaly detection algorithms can analyze vast amounts of system and driver data efficiently. And they can perform this analysis using additional data types and in far greater quantities than traditional methods can handle.
For example, during the manufacturing phase, the use of image data as an input for root cause analysis helps organizations correlate failure modes to possible flaws in the underlying manufacturing procedures.
With issues arising in the field, text recognition and Natural Language Processing enable the inclusion of service provider notes in the analysis process. Each of these approaches can reveal very specific root causes months faster than traditional analysis — and oftentimes diagnose issues that may not be uncovered any other way.
Machine learning can provide far more precise and — importantly — evolving maintenance recommendations to help drivers protect their vehicle investment as well as their safety. Rather than a static maintenance schedule that gets updated a few times a year, a predictive analytics model can continue to learn from thousands of performance data points collected from manufacturing plants, suppliers, service providers and actual vehicles on the road. The industry is well on its way to completely customized maintenance schedules that evolve over time to be increasingly more tailored to individual drivers and vehicles, and can even adapt to changing conditions and new performance information.
Predictive maintenance helps increase customer satisfaction and brand reputation, while also improving compliance with recommended maintenance. It can also be a source of additional revenue for car makers as an added-value service.
Note: The same technologies enable predictive maintenance for fleet management, saving on major repairs and protecting the ROI on each vehicle. Predictive maintenance can also help keep manufacturing systems working at optimal performance levels — protecting yield, helping to ensure quality and safety, and ultimately saving time and money.
Throughout the supply chain, analytical models are used to identify demand levels for different marketing strategies, sale prices, locations and many other data points. Ultimately, this predictive analysis dictates the inventory levels needed at different facilities. Data scientists constantly test different scenarios to ensure ideal inventory levels and improve brand reputation while minimizing unnecessary holding costs.
After analyzing the gap between current and predicted inventory levels, data scientists then create optimization models that help guide the exact flow of inventory from manufacturer to distribution centers and ultimately to customer-facing storefronts. Machine learning is helping parts and vehicle manufacturers — and their logistics partners — be more efficient and profitable, while enhancing customer service and brand reputation.
The automotive sector is nothing if not competitive. Machine learning and data science are the new frontier, enabling organizations to discover and harness hidden value in their operations — and create new opportunities for growth. The open source community is the engine of innovation across most of data science, which is why automotive executives would be wise to embrace a platform that leverages innovation from open source. Cutting-edge open-source software packages and libraries in a centrally managed, enterprise-class data science platform enable data science teams to do more than just bolt on various point solutions. They can collaborate, learn and evolve to address thousands of use cases with just one platform.
Talk to one of our experts to find solutions for your AI journey.