Introducing Anaconda’s Maker Blog Series!
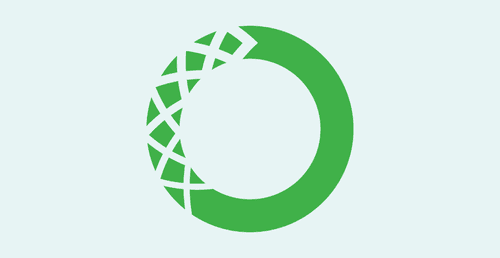
Kelly Davis-Felner
3min
Every year, we conduct a survey to assess the pulse of the data science community and to better understand the responsibilities, expectations, and challenges of one of the world’s most sought-after professions. From February 12 to April 20 of this year, we asked individuals using data science and machine learning tools, as well as those assisting data scientists with their work, and received responses from a total of 2,360 people. Respondents included data scientists, researchers, developers, analysts, data engineers, business managers, and more, showing that the disciplines and skill sets that power the field of data science remain as diverse as ever.
Data science has matured beyond its infancy and entered into its adolescence. Since the term “data science” was coined in 2008, we’ve witnessed an explosion of new tools, applications, and startups in the field, resulting in a crowded landscape where it’s easy to be preoccupied by the shiny and new. However, we’re beginning to see a shift in that attitude, as more and more industry players demonstrate a heightened understanding of data science’s capabilities, limitations, and concrete value. Across industries, there’s a push to view data science more as a strategic business function rather than a novelty or sci-fi gadget.
To review the full findings on the 2020 State of Data Science, we invite you to download the report here.
Setting data science up for success
The role of the data scientist has manifested in different ways across organizations. Among the data scientists who responded to our survey, 28% work in a data science Center of Excellence (COE), while 22% work in R&D, and 21% work in a line of business. It’s clear that there is no one-size-fits-all approach to team structure.
But it is notable that team structure correlates with employee satisfaction and success. For example, respondents working in IT reported less effectiveness in demonstrating data science impact than their counterparts working in other team structures. The Center of Excellence model, often found in more data-mature companies, emerged as an approach most strongly correlated with demonstrated business value, with over 70% of respondents working in a COE reporting they were “mostly” or “almost always” effective in demonstrating impact.
The need for cross-functional visibility
Across survey respondents, there were several instances where we found a marked difference in sentiment based on role. Take open-source security, for example. Business managers and system administrators were found to be more concerned than average about managing security and vulnerabilities in open-source tools, whereas researchers and data scientists reported the lowest levels of concern. Given that 30% of respondents with knowledge of their company’s security practices stated their company did not have any mechanism to secure their open-source data science pipelines, it’s important that data science teams communicate the risk involved from the outset. The observed discrepancy suggests an opportunity for further cross-functional visibility into each role’s concerns and challenges so that teams work together in lockstep.
Anaconda’s VP of Services Michael Grant and I discuss these themes in a webinar reviewing the survey results in more depth. Join us to hear our discussion by signing up here.
What’s to come
This year for the first time, we asked respondents to tell us what they consider to be the biggest issue in AI and machine learning. Data bias and privacy impacts were rated as top of mind for almost half of all respondents. It’s clear that challenges related to data bias and privacy are having a profound effect on businesses, society, and our personal lives, and data professionals are rightfully concerned about these implications.
But there’s a disconnect. Our study found that only a minority of organizations have implemented solutions to address either data bias (15%) or model explainability (19%). Furthermore, only 15% of universities indicated that they offer training in ethics for data science. While we are thinking about pressing issues of data ethics and bias, there remains a gap in how we’re taking steps to address them. It’s important to continue having these conversations and to consider all aspects of the pipeline, from education through to enterprise oversight, where we should pay close attention to these issues.
To that end, our CEO Peter Wang will be sitting down with Danielle Oberdier, founder of DiKayo Data for a discussion of fairness in AI. Join us for the discussion by signing up here.
Data scientists are poised to step up and help drive strategic transformation in their organizations. Tools and roles are evolving, and we’re increasingly engaging in conversations around how data science can improve our lives. We’re optimistic that the data science industry is on the right track for open growth.
Talk to one of our experts to find solutions for your AI journey.