New Release: Anaconda Distribution 2022.10
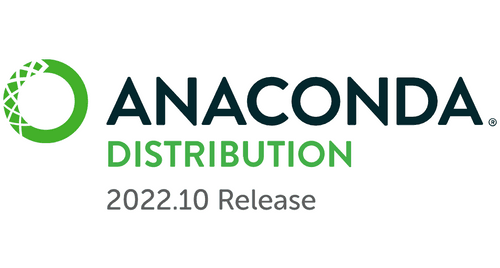
The start of a new year is a popular time to recalibrate habits and set goals for improvement, both personally and professionally. First, it’s helpful to take stock of where things stand, in order to hone in on potential areas for betterment. In data science, the past year represented another step forward in the maturation of the discipline, especially with the onset of the COVID-19 pandemic. We saw researchers come together to harness the power of data and open-source software for public health, and concepts of statistical modeling become mainstream as we looked to curb the disease’s spread. At the same time, we had a glimpse into a future of division if we don’t pay heed to issues of bias in data and explainability in algorithms.
Data scientists have a responsibility to guide the ongoing data-driven transformation of the organizations they work in. We must all ask ourselves: how do we double down on the good and overcome bad habits and practices? For data science practitioners of all skillsets, I’d like to suggest the following resolutions. I hope that these thoughts will empower data scientists to lead the way forward in this dynamic field.
1. Deepen your subject matter expertise
Half the process of problem-solving is identifying the problem you’re trying to solve. For data scientists, this requires deepening our familiarity with the domain of a given problem and not concentrating exclusively on the “data science” components. Continue absorbing information from the subject matter experts and line-of-business managers around you so that you can further refine your approach and applications. The most sophisticated algorithm won’t be of value if it’s not fitted for the domain at hand.
2. Align on security best practices
Security is never one-and-done. It’s a continuous practice that all data scientists should take seriously—especially when it comes to the open-source tools that may fly under the radar of traditional IT security. Because open source is such an integral part of the data scientist toolkit, it’s crucial that practitioners keep up with patches, updates, and vulnerabilities of their tools. Take the time at the start of the year to touch base with IT counterparts and align on an approach that will allow for speed in experimentation without increasing security risk vectors.
3. Contribute to open source
There is no shortage of support needed in the open-source community, whether you’re identifying bugs, writing documentation, or proposing features. Open source is an ecosystem of collaboration that transcends geographical locations, time zones, and organizations—take time to engage with the community that has brought us so many of today’s integral backend technologies, while also expanding your professional network and learning new skills.
4. Never stop learning
The skills needed to succeed in data science may seem overwhelming at times—the ability to not only code, but also grasp and apply abstract mathematical concepts and theorems. At the same time, the beauty of data science is that there’s always something new to learn. One of the best ways I recommend data scientists to do this is to read other peoples’ code on Kaggle and Github. Understanding why certain decisions were made about algorithms or packages will expand your mindset on what kind of tools you have at your disposal. In that vein, make sure to also write reliable documentation for your own code to help others learn the “why” behind your decisions as well.
5. Find your community
The data scientist role can feel lonely at times because many practitioners are either one of only a few in their organization or they sit dispersed across teams. Because the data science space is evolving so quickly, being plugged into the community is crucial to understanding best practices and sharing resources. While COVID-19 paused in-person meetups, I encourage all data practitioners to find and join the vibrant groups that have grown up around their favorite libraries or languages. Last year, we surveyed over 2,000 data practitioners and found that while an overwhelming majority were interested in finding a mentor, more than half didn’t know where to look for one. Offer support and mentorship where you can—we’re all figuring out this changing field together!
There’s no time like the present to start anew—especially if it’s the beginning of the year. As we dive into day-to-day work and delivering on our goals, I advise data practitioners to keep these resolutions in mind. Given the constant innovation in our fields, we should all commit to our ongoing learning, our community, and the security of our tools.
Talk to one of our experts to find solutions for your AI journey.