Anaconda Brings Data Science to Linux on IBM Z and LinuxONE
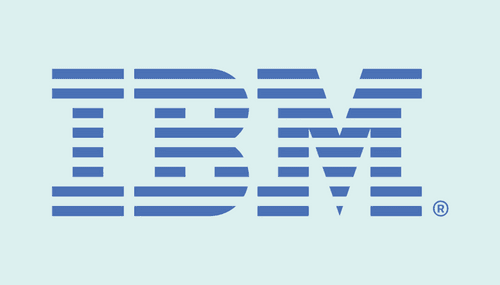
By Elizabeth Winkler
Another Strata conference has come and gone. We had an incredible time meeting with a huge number of Anaconda users who came by our booth to chat! We also noticed some really interesting trends when it comes to the future of data science, machine learning, and AI.
Since the launch of Kubernetes into the Cloud Native Foundation three years ago, talks of Kubernetes eating the container world have only increased in the context of DevOps. Kubernetes has become the de facto for organizations looking to stand on the shoulders of (Google) giants to achieve a fully resilient and scalable infrastructure.
The natural fit between Kubernetes and the AI space is undeniable. At Anaconda, we saw this trend early on and predicted that the ML space would follow suit, as a cloud native infrastructure is a natural fit for doing machine learning at scale. This is why Anaconda Enterprise was built on Docker and Kubernetes by default, and it seems that the rest of the industry has caught on.
There were a number of Kubernetes-centric talks at Strata this year, all of them jam-packed. In fact, our own Mathew Lodge’s talk was so popular it left a number of attendees lined up outside the at-capacity room, unable to enter. Noticing the demand, the terrific conference organizers gave Mathew an encore slot—in a bigger room—the following day.
The message is clear: if you want to run machine learning models at scale, you need to get on board with Kubernetes.
If Kubernetes stole the show on the software side, GPUs certainly stole the show on the hardware side. We saw nearly as many sessions about leveraging GPUs to accelerate machine learning as we did about Kubernetes. In fact, a number of sessions combined both topics in an ultimate buzzword explosion.
As we know, success in machine learning comes from moving through the data science lifecycle as quickly as possible. GPUs are a great way to train models on ever increasing amounts of data at a speed that was unimaginable just a few years ago. Anaconda is well ahead of this trend by working closely with NVIDIA and Cisco to make it very easy for teams to get the most of their GPU clusters.
If a model falls in a forest…
Someone very wise at AnacondaCON 2018 gave a great quote: “If your model isn’t deployed into production, does it really exist?” While a non-productionized model may exist, it’s certainly not adding much value to your business.
Attendees at Strata were very interested in learning from peers who have been successful at extracting value from their data teams by getting models into production. Sessions led by the companies well known for their ability to productionize ML were particularly well attended.
Data science is out; machine learning and AI are in.
It was hard not to notice the huge shift in messaging from all the vendors. Data science is still present, of course, but everyone is now aspiring to own the ML/AI space. With that being said, the jury is still out on ML vs AI and how often each appears on taglines. Which term do you prefer?
Talk to one of our experts to find solutions for your AI journey.