I Have an Anaconda Nucleus Account – Now What?
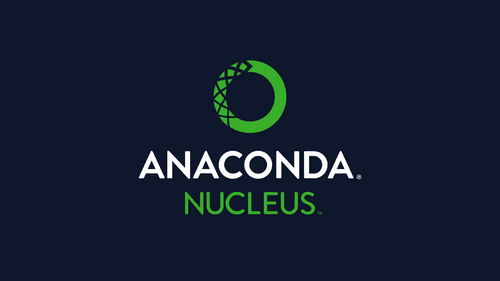
The over 45 speakers at AnacondaCON 2019 delved into how machine learning, artificial intelligence, enterprise, and open source communities are accomplishing great things with data — from optimizing urban farming to identifying the elements in stars to developing strategies to preserve privacy. Joined together by their love for robust technologies that enable them to bring order to complex data, the speakers showed that their diverse work was strengthened by their shared attention to the humanity involved in collecting, using, and protecting data. Dr. Bethany Doran kicked off the Artificial Intelligence and Machine Learning track with her talk, “The Future of Data, Humanism, and Health: A Physician’s Perspective.” She cited the significant amount of health data available, which is estimated to double every 73 days by 2020 (IBM Healthcare). The sheer amount of data isn’t sufficient for successful analysis, though. She likens this raw data to gold ore that requires experts to transform it into gold — something meaningful and able to influence people’s lives. As a physician, being able to gather data during interpersonal engagement alongside artificial intelligence provides a critical combination for informed and careful data collection. Like Dr. Doran’s note on the value of gathering data through interpersonal connections, Dr. Marianne Hoogeveen’s talk, “Plant Factory: Data-Driven Indoor Farming at Scale” also addressed the value of pairing manual interventions with AI. Highlighting her work at Bowery Farm, she discussed the intricate work of managing temperature, location, lighting, and growth cycles using AI. But, she notes, there comes a time when human judgments must be made in order to augment the benefits of automated systems. While there are positive aspects to incorporating human judgment, several speakers stressed the importance of checking biases at each stage of research — from setting parameters to analyzing the results. As Dr. Doran stated, “If you’re unaware of your biases, you need to be thinking about what to include or exclude in algorithms and see how they influence the stories you can tell.” Dr. Natalie Hinkel’s research into the elemental composition of stars reaffirmed that even observing natural occurrences requires a critical attention to how biases can shape data gathering and analysis. Self-awareness about biases in one’s research is critical, but what if you want to highlight, fight, and prevent biases in the world? Dr. Gaurav Sood shared his research on how to use AI to analyze names, to identify race and ethnicity. He explored how this can be leveraged to study biases in media coverage, fairness in lending practices, and political accountability — while acknowledging the risk of how this data could potentially be misused. One way to address the risk of bias impacting your own work is to embrace transparency. John Miller’s talk, “Getting the Green Light for your ML Model,” emphasized the importance of knowing your audience and building transparency into the model-development process. He encouraged the audience to seek input from the open source community in order to provide more “brain-friendly models” that are easy for people to understand. Transparency with data becomes even more critical when thinking about the ethical components of who owns data about whom. Dr. Doran provided the example of patients who believe they’ve experienced a shock from their pacemaker. Because the pacemaker company owns that data, neither the patient nor the physician are able to confirm whether a shock occurred without performing a series of tests. This stifles innovation and creates significant hurdles for patients and physicians. One answer to this is to keep datasets open source as much as possible (while balancing the need for privacy) to develop better algorithms. While many speakers addressed the importance of keeping datasets open, there was also an emphasis on the importance of privacy. The medical community especially recognizes this need, but all datasets about people have the risk of leaking crucial information. Dr. Stephen Bailey’s talk on “The Data Scientist’s Guide to Preserving Privacy” delved into these risks and provided guidelines on how to ensure private data is protected. According to Dr. Bailey, “data subjects are the ultimate owners of their data and retain rights, including the right to erasure, right to be informed, and the right to restrict processing.” Specifically, those working with datasets are not data owners but are “data stewards.” As privacy is more protected — through practices such as masking, incorporating noise, and leveraging K-anonymization — data may become more homogenous. This tradeoff between the risks of leaking private data and losing specificity has to be balanced, which requires careful attention to how datasets are handled from the beginning. While these talks spanned sectors and leveraged a variety of technologies, uniting them was a clear attention to the roles that interpersonal connections, bias, transparency, and privacy play when working with data.
Talk to one of our experts to find solutions for your AI journey.